Boris Kontsevoi is a technology executive, President and CEO of Intetics Inc., a global software engineering and data processing company.
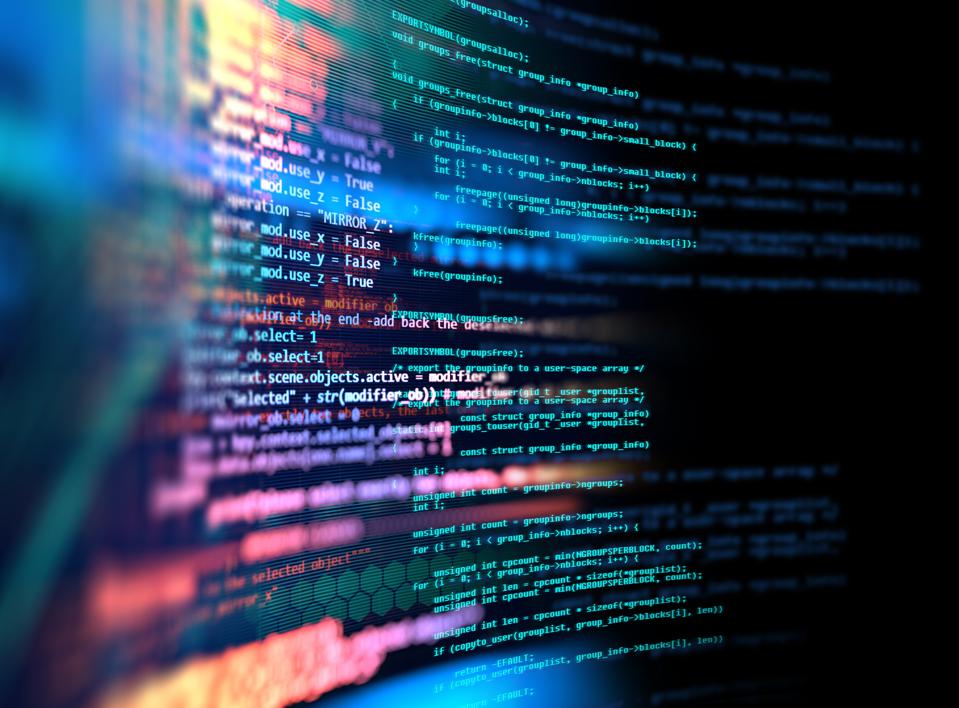
getty
Many of today’s emerging technologies and products heavily rely on artificial intelligence (AI) and machine learning (ML). And while there are hundreds of articles written about this topic, very few get into the nitty gritty of what truly powers AI: data.
The definition of artificial intelligence varies depending who you ask. A data scientist will have a much different answer than someone who is just peripherally aware of AI. Even within the field of data science, there’s debate about what exactly AI means. And depending who you ask, AI can be a good or bad thing. Some scientists see it as an important tool in the fight against cancer and the exploration of space while others hear the words “artificial intelligence” and conjure up images of robots taking over the world. In my opinion, AI is pivotal technology that can—and has—helped us accomplish many things.
What does AI truly mean? The definition is actually quite simple: the science of training computers to do human tasks. This is the most basic definition and also the oldest, dating back to the 1950s when computer scientists Marvin Minsky and John McCarthy began researching AI.
In modern times, AI’s definition has expanded to include more specificity. For instance, Francois Chollet, an AI researcher at Google, thinks AI is specifically tied to a machine’s ability to adapt and improvise in a new environment. It also includes the ability to generalize its knowledge and utilize it in unfamiliar scenarios. “Intelligence is the efficiency with which you acquire new skills at tasks you didn’t previously prepare for,” he suggested in a podcast recorded in 2020. “Intelligence is not skill itself, it’s not what you can do, it’s how well and how efficiently you can learn new things.”
MORE FOR YOU
Though AI and machine learning (ML) are oftentimes used interchangeably, in reality ML is a scientific field, a tool that makes AI happen. ML models look for patterns in data and try to draw conclusions, i.e. they train a machine how to learn. This leads me to the most basic part of AI and ML: data. And to be even more specific: datasets. Every single AI application requires a suitable dataset.
Datasets for machine learning are the main commodity in the world right now. Everybody is talking about AI and AI applications but a few are focusing on how accurate the data is and if the data is actually correct. Data collection needs to be deliberate—the success of its intended application depends on it.
As those in data science know, datasets are necessary to build a machine learning project. The dataset is used to train the machine learning model and is an integral part of creating an efficient and accurate system. If your dataset is noise-free (noisy data is meaningless or corrupt) and standard, your system will be more reliable. But the most critical part is identifying datasets that are relevant to your project.
So your company has decided to make the jump into data science and needs to collect data. But if you don’t have any, where do you start? The answer is twofold. One option is to rely on open source datasets. Companies like Google, Amazon, and Twitter have a ton of data they’re willing to give away. And many online sites dedicated to AI and AI applications have compiled free categorized lists which make finding a good dataset even easier. Wikipedia has a fairly comprehensive list of available datasets too.
There are some things to keep in mind as you begin searching for the ideal open source dataset for your system:
• Pursue clean datasets. It’s easier overall if you don’t have to spend time cleaning the data yourself.
• Depending on the scale of your project, search for datasets without a lot of rows and columns. The fewer the rows, the easier it is to work with.
• And perhaps the most important part of your dataset hunt: There needs to be an interesting discovery within the dataset.
The other option is to mine your own data from internally collected records of your company. Knowing the problem you’re trying to solve is crucial in the discovery phase and will help decide which data may be more valuable to collect. It’s also important to remember that data collection by humans is oftentimes tedious and employees most likely won’t be excited about doing manual data entry. Instead, consider using robotic process automation systems. RPA systems are basic bots that can do repetitive and mundane tasks.
I’m guessing you’ve heard the term ‘big data’ thrown around. Who hasn’t? It’s one of this decade’s most popular terms. But if your company is just dipping its toe into AI and ML, it’s better to stick to smaller and less complex datasets. You can tackle big data once you’ve mastered a smaller scale ML system.
What we can do—and what we’ve already done—with AI and AI applications is incredible. But there are still some major limitations and challenges. As research firm McKinsey & Company summarizes: “While much progress has been made, more still needs to be done. A critical step is to fit the AI approach to the problem and the availability of data. Since these systems are “trained” rather than programmed, the various processes often require huge amounts of labeled data to perform complex tasks accurately. Obtaining large data sets can be difficult. In some domains, they may simply not be available, but even when available, the labeling efforts can require enormous human resources.”
AI and ML are two of the most important scientific breakthroughs in recent history. Both will continue to enhance emerging technologies and influence robotics and the Internet of Things (IoT) in the future. We’ve made enormous strides in the science of AI—and datasets—over the past 10-20 years and we’ve only just scratched the surface.
Forbes Technology Council is an invitation-only community for world-class CIOs, CTOs and technology executives. Do I qualify?