It’s hard enough for people to categorize or discuss art, but it’s even more difficult for artificial intelligence. Several research groups have recently tried to apply machine learning to large databases of artworks to sort and describe them in a meaningful way.
First, researchers from Zhejiang University of Technology in Hangzhou, China, compared different neural networks to find out how well they perform at art classification. They used images from WikiArt and other digital collections to train the neural networks to learn what images of a certain art style look like. Then they asked the different neural network models to identify the art style of other images.
This is quite a tricky task, even for humans. Some art styles are easy to recognize from the way the image is created. Learning which artworks fall under the cubism genre wasn’t a problem for the neural networks. But some genres are quite similar to each other and occurred around the same time. That made it difficult for the programs to learn which is which.
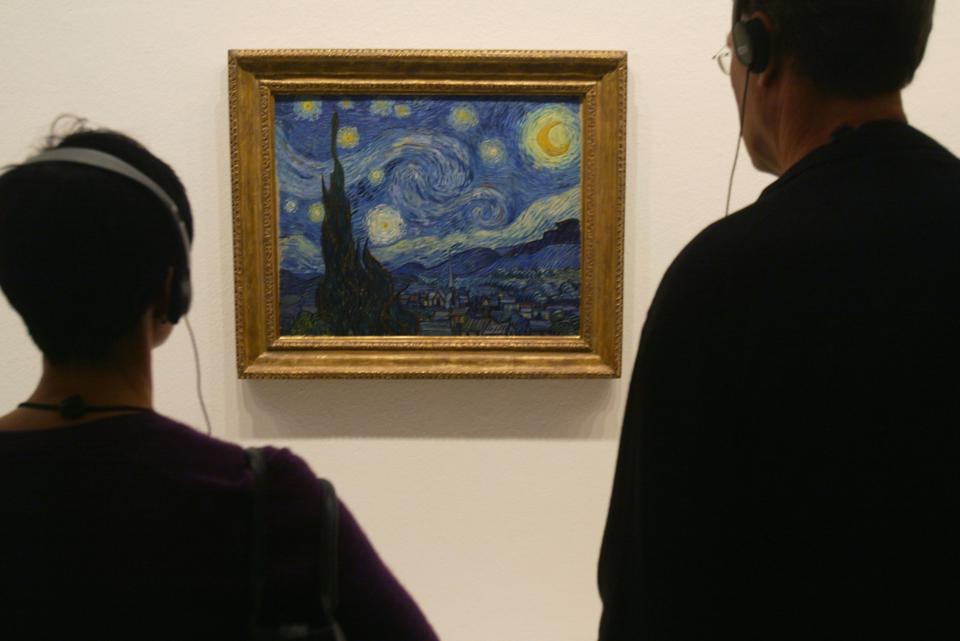
Vincent van Gogh’s “Starry Night”, seen here at an exhibit in Berlin, Germany, was one of the images … [+]
Getty Images
The art classification neural networks also had trouble with tasks that humans wouldn’t find very difficult at all, such as understanding the difference between cityscapes and landscapes. The difference between buildings and nature is obvious to us, but to a computer, they both look like images with similar elements of “outside”. It doesn’t have a way of knowing that the clouds and sky in these images are not the key defining factor of these two categories.
For human art lovers, learning which style or category a piece of art falls in is a relatively straightforward and objective task. Like the neural networks, we can learn how to do that by looking at a lot of art and finding patterns. But there’s something humans do that computers don’t: we also form opinions about the art and can share in words how looking at it makes us feel. Computers can’t do that yet – or can they?
MORE FOR YOU
Artificial intelligence is only as good as its training data, so to be able to teach an AI to form opinions and emotional statements about art, you need a vast collection of human-created descriptions of different artworks. That’s exactly what researchers from Stanford University, Ecole Polytechnique and King Abdullah University of Science and Technology have done. They created the ArtEmis dataset which includes over 400 thousand emotional attributes and descriptions for over 80 thousand images indexed in WikiArt.
To create ArtEmis, the team asked volunteers to share their main emotion about an artwork, and to explain that in a sentence. As you would expect, people’s reactions varied widely. One person might find a painting of a field peaceful while someone else finds it slightly ominous. In fact, having both positive and negative reactions to the same painting was so common, this happened to 61% of the images in the ArtEmis database.
So what does an AI make of all those human descriptions of art? When trained on the ArtEmis dataset, different systems started creating their own captions for given artworks. Some of them were very convincing, but others missed the mark. AI-generated descriptions of Rembrandt’s painting “The Beheading of John the Baptist” included “the woman looks like she is having a good time” and “the man in the middle looks like he is in pain”. Any human would recognize these descriptions as complete nonsense (or at the very least a major understatement) considering the scene in the painting.
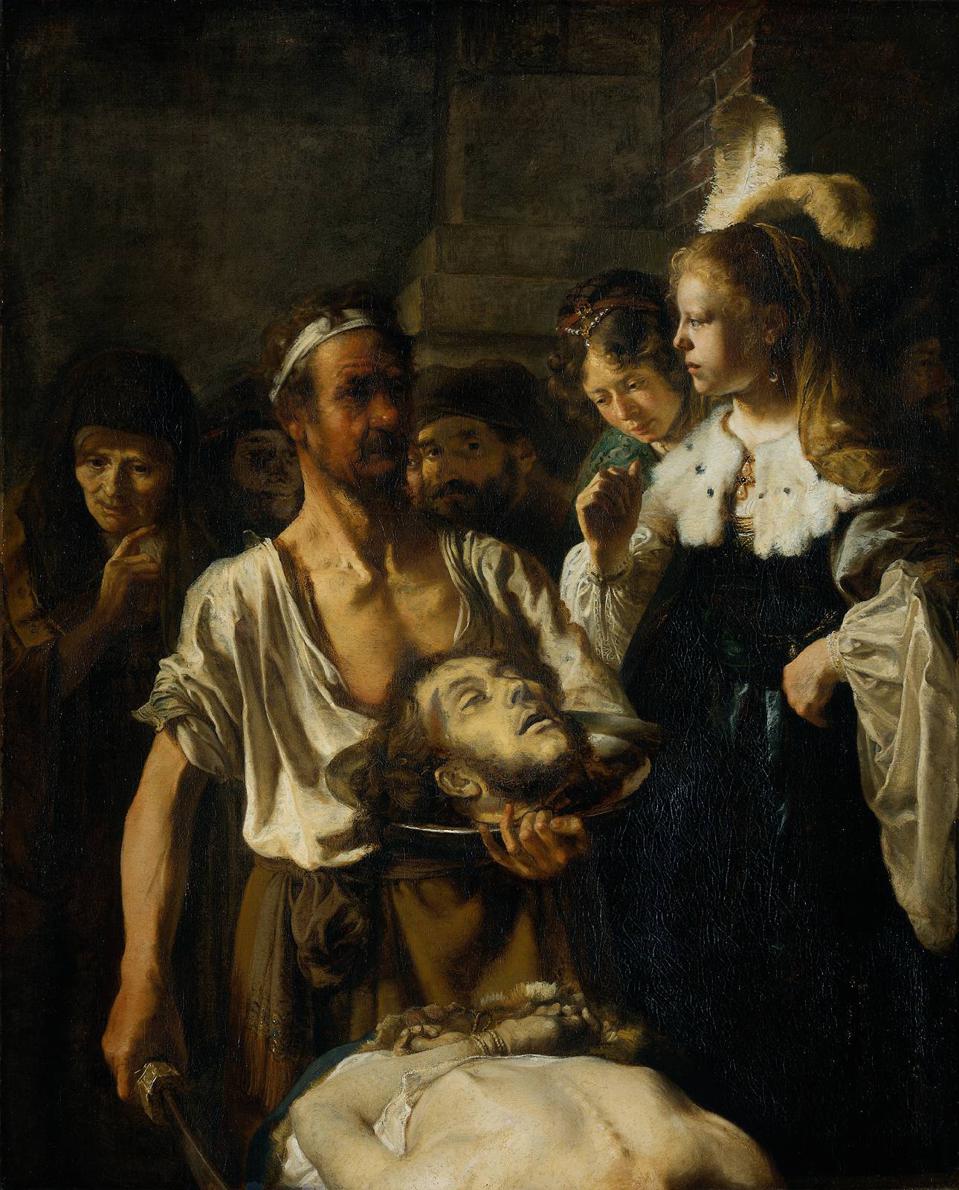
An AI algorithm creating descriptions of images based on replicating human emotion said of … [+]
WikiArt / public domain
About half of the computer-generated descriptions passed the Turing test, which means that AI’s can indeed learn to create new (and believable) descriptions of art, but it’s still far from perfect. That’s not surprising, considering it’s already a challenge to teach an AI whether a painting is a landscape or a cityscape.
Art can be hard to classify and people’s opinions about paintings are highly subjective, which makes it even harder for artificial intelligence to understand the patterns of our classifications and descriptions. But the experiments done in these two new studies show that computers are getting better at these tasks. Humans are still better at categorising and describing art, but AI programs are learning quickly.