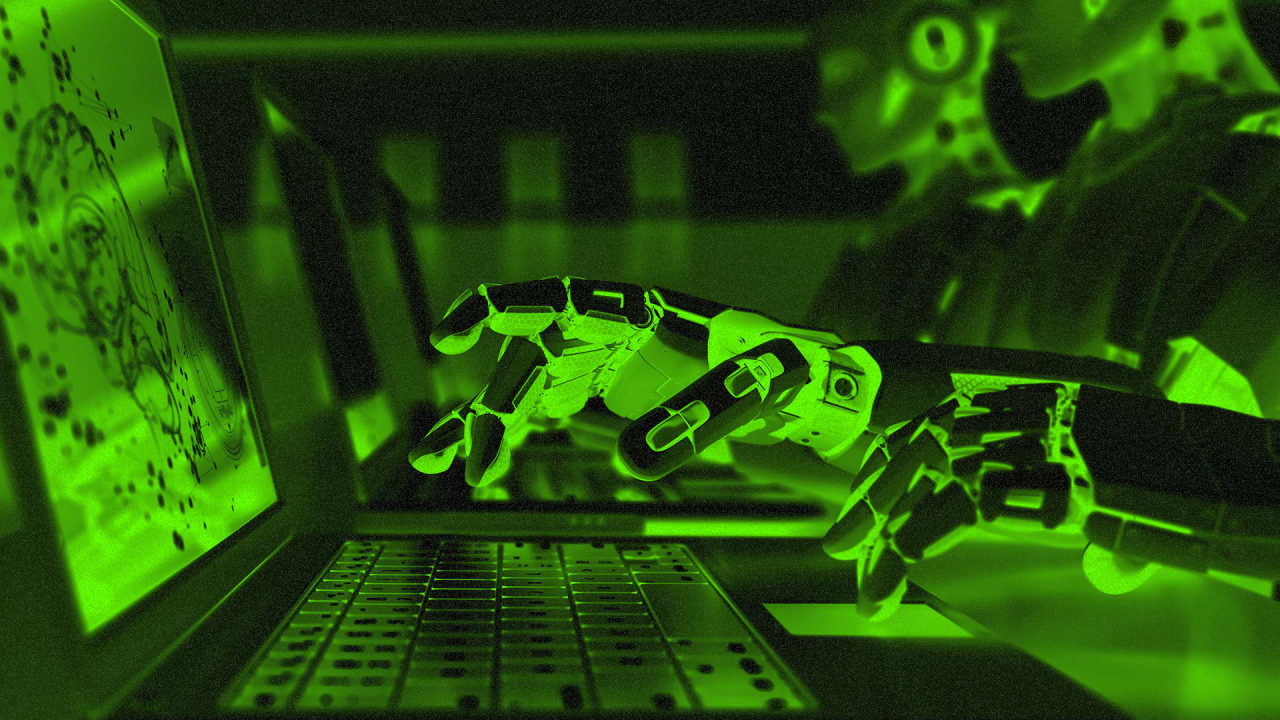
By Jeffrey Funk and Gary N. Smith 9 minute Read
In 1965, Herbert Simon, who would later be awarded the Nobel Prize in Economics and the Turing Award (the “Nobel Prize of computing”), predicted that “machines will be capable, within 20 years, of doing any work a man can do.” In 1970, Marvin Minsky, who also received the Turing Award, predicted that, “in from three to eight years we will have a machine with the general intelligence of an average human being.”
The implications for jobs were ominous, but robotic-takeover predictions have been in the air for a hundred years, ranging from Karel Čapek’s 1920 play R.U.R. (Rossum’s Universal Robots) to Daniel Susskind’s 2020 award-winning book, A World Without Work. Add in Elon Musk, who always seems to have something to say: “What’s going to happen is robots will be able to do everything better than us . . . all of us. . . . When I say everything—the robots will be able to do everything, bar nothing.”
We are reminded of two sayings:
A Danish proverb states “it is difficult to make predictions, especially about the future.”
Ed Yardeni said (about predicting stock prices): “If you give a number, don’t give a date.”
Yet cocksure researchers are blithely using computers to predict which jobs will be taken over by computers. How could computer algorithms, which literally do not know what words mean, possibly know which skills are required to succeed in a job and whether computers have these skills? Computers can look for statistical patterns, but cannot tell whether the discovered patterns are meaningful or meaningless.
Computer algorithms will have a devil of a time predicting which jobs are most at risk for being replaced by computers.
This is how an Amazon algorithm for evaluating software engineer job applicants ended up discriminating against women. The algorithm could not assess job skills so, instead, it looked for keywords in résumés and—since there were few women in Amazon’s technical-job résumé database—the algorithm assumed that applicants who went to women’s colleges or participated in female activities such as women’s tennis or women’s singing groups were not good software engineers.
The more general point is that computer algorithms will have a devil of a time predicting which jobs are most at risk for being replaced by computers, since they have no comprehension of the skills required to do a particular job successfully.
Assisting humans is easier than replacing them
In one study that was widely covered (including by The Washington Post, The Economist, Ars Technica, and The Verge), Oxford University researchers used the U.S. Department of Labor’s O∗NET database, which assesses the importance of various skill competencies for hundreds of occupations. For example, using a scale of 0 to 100, O*NET gauges finger dexterity to be more important for dentists (81) than for locksmiths (72) or barbers (60). The Oxford researchers then coded each of 70 occupations as either automatable or not and correlated these yes/no assessments with O*NET’s scores for nine skill categories. Using these statistical correlations, the researchers then estimated the probability of computerization for 702 occupations.
There are two glaring problems with this study. First, the Oxford group’s yes/no labeling of an occupation as being automatable is far too simplistic. For many (most?) occupations, computers can be invaluable assistants, but cannot replace humans fully. Lawyers can use computers to search for case precedents, but cannot rely on computers to make persuasive arguments. Meteorologists can use statistical programs to make weather forecasts, but cannot rely on computers to specify the variables that should be used in such models. Writers can use word processing programs to format their work and avoid spelling mistakes, but cannot rely on computers to write compelling novels.
Second, if the O*NET assessments in nine skill categories were sufficient, it would be relatively easy to predict the best job for every person and to predict how well any person would do in any job. It is not. Anyone who has ever worked for someone, worked with someone, or had someone work for them (did we leave anyone out?) understands how difficult it is to know in advance whether someone will be a good boss, coworker, or employee.
Some important skills are difficult to measure; others may be overlooked. For example, a robot with excellent finger dexterity won’t be a good dentist if its image-recognition software is bad at recognizing cavities. Radiology AI’s struggles are not comforting. Similarly, you may be in for a surprise if you trust a robot to cut your hair simply because it can open and close scissors.
A 2019 Stanford University study used the overlap between the text of robot, software, and AI patents and the text of job descriptions to estimate the impact of robots, software, and AI on jobs. Stanford’s study implies huge job losses in finance, insurance, real estate, engineering, and other white-collar occupations, particularly service workers, yet no such losses have shown up in employment statistics. Most job losses have been limited to secretaries, bookkeepers, and data entry workers—and one doesn’t need a textual analysis of anything to have predicted that.
A 2021 study published in the Strategic Management Journal, the flagship publication of the Strategic Management Society, relied on crowdsourced survey data from gig workers to link common AI applications to occupational abilities. One of their striking conclusions was that surgery and meat slaughtering are very similar occupations, but surgeons are more at risk of being replaced by robots because their jobs require more intelligence!
Computers do not now and are not likely to soon have any of the cognitive abilities required of surgeons.
Both require deft physical manipulation of human or animal tissue. Although the occupations require similar physical abilities, such as manual dexterity, finger dexterity, and arm–hand steadiness, the occupations’ measures suggest that surgeons are far more exposed to AI than slaughterers. The measure for surgeons is at the 52nd percentile in relation to other occupations in our sample, while the measure for slaughterers is at the second percentile (indeed, it is the 10th least-exposed occupation). The difference between the measures in these two occupations seems to arise from the cognitive abilities required by each occupation.
Although the two occupations require similar physical abilities, a number of cognitive abilities related to problem solving, such as problem sensitivity, deductive and inductive reasoning, and information ordering, are highly important for surgeons but not for meat slaughterers.
When we read that paragraph, we each read it again to make sure that we hadn’t missed a not or other word that would completely reverse the conclusion. Nope, they really meant it, evidently because of the fundamental misconception that computers are more intelligent than humans. Computers do not now and are not likely to soon have any of the cognitive abilities required of surgeons. The reason that robots perform better than humans on assembly lines is not because they are smarter, but because the work is tiring, boring, and mindless.
A doctor with more than 40 years of experience wryly told us that, “they state surgeons are smart and the work they do is complex [and] smart, but AI is very smart, smarter than humans. Butchers are dumb hackers, so our AI can’t go down the cognitive scale to become dumb enough to replicate their work.” Personally, we would rather have a robot cut a steak for us than cut open our bodies.
You can’t automate common sense
The reason AI has not replaced radiologists is precisely because the algorithms do not have the cognitive abilities needed to do a good job. A 2021 study of 2,212 machine learning models for the diagnosis or prognosis of COVID-19 from chest radiographs and CT scans concluded that, “none of the models identified are of potential clinical use.” Another 2021 study found that the algorithms’ search for distinguishing COVID-19 characteristics often focused on systematic differences around the borders of the x-ray images; for example, differences in patient position, x-ray markings, radiographic projection, or image processing.
Another 2021 study demonstrated that an AI algorithm was seemingly able to identify the presence of COVID-19 even when the lung images were removed from the x-rays! The algorithm evidently noticed patterns in the outer borders of the images that happened to be correlated with the presence or absence of genuine COVID-19 pathology—which meant that the algorithm was completely useless for analyzing new images.
The fundamental reason why most jobs are safe from robots is that computers do not have the common sense, wisdom, or critical thinking skills required to do a good job.
The other gross mistake made by robot-takeover studies is the neglect of the cost-benefit calculations made by employers. More than half a century ago, studies of technology diffusion made by Zvi Grilliches and Edwin Mansfield confirmed the obvious—technology will be adopted more quickly when the economic benefits far outweigh the costs. Unless a job is robot-proof, it’s all about the money. Yet, none of these three studies of robotic takeover consider either the costs or the benefits!
A commonsense approach is to look for evidence of these costs and benefits in the diffusion of robotics, AI, and other technologies. For robots, the 293,000 installed robots in the U.S.—far fewer than the millions of manufacturing jobs that still exist and that were lost over the last 50 years—suggest that the economics of robots have never been as good as proponents claim.
For AI, its diffusion began in the virtual worlds of advertising, news, finance, and e-commerce, building on top of the benefits previously provided by data analytics and software automation, whose usage also probably reduced the cost of implementing AI. These trends will likely continue with each new addition of AI, building not only on machine learning advancements, but also from incremental advances in robotics and other complementary technologies such as virtual and augmented reality, the Internet of Things, and drones.
Business-school academics love mathematical models because they seem scientific, even though most real-world business decisions involve judgements weighing complex costs and benefits that are difficult to measure. For too many academics, AI is just the next logical step and, not coincidentally, a confirmation of the way academics do things. It’s about turning the data over to computers and letting them number-crunch their way to good decisions. As Upton Sinclair said almost a century ago: “It is difficult to get a man to understand something, when his salary depends on his not understanding it.”
Is your job robot-proof? You don’t need professors—or algorithms—to tell you the answer. All you need do is consider whether it requires common sense, wisdom, and critical thinking skills.
Jeffrey Funk is an independent technology consultant who was previously an associate, full, or assistant professor at National University of Singapore; Hitotsubashi and Kobe Universities in Japan; and Penn State where he taught courses on the economics of new technologies.
Gary N. Smith is the Fletcher Jones Professor of Economics at Pomona College. He is the author of The AI Delusion and coauthor (with Jay Cordes) of The 9 Pitfalls of Data Science and The Phantom Pattern Problem.