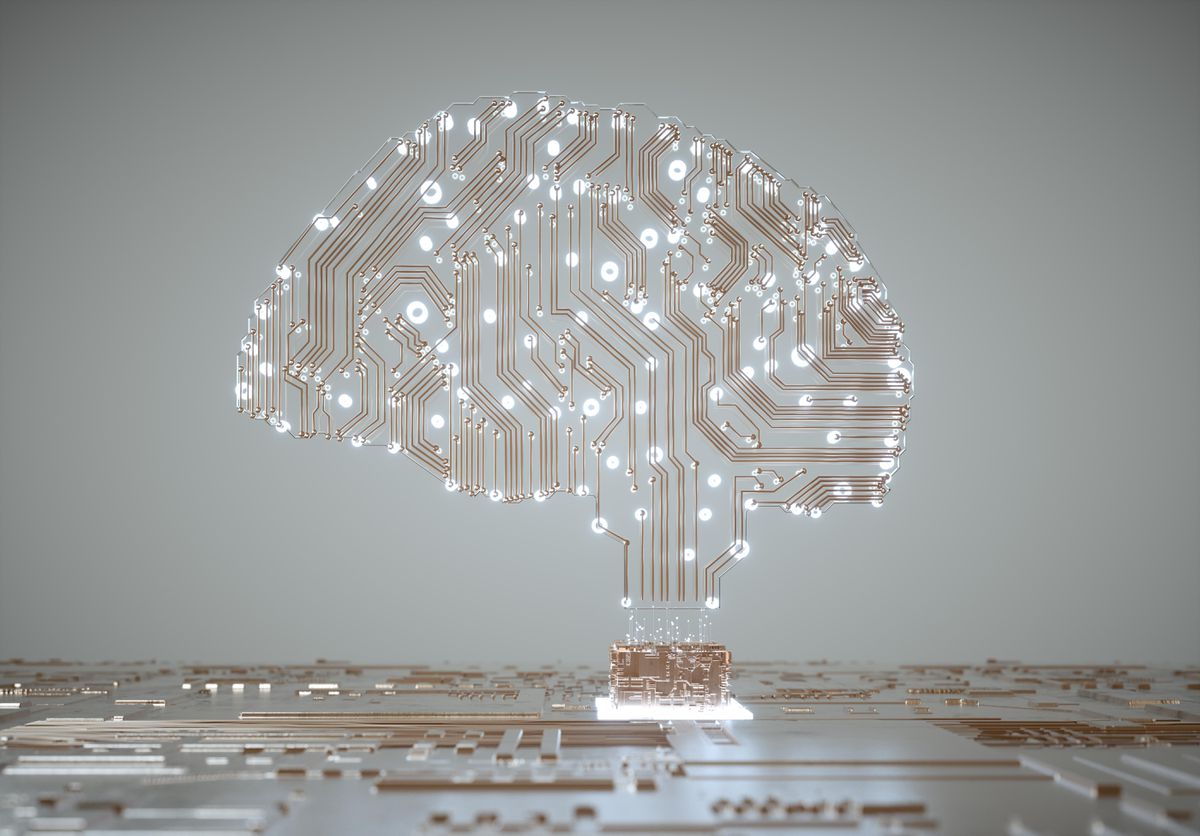
Getty
In the science fiction canon, the rise of the machines comes swiftly and visibly. After the initial development of primitive AI systems, the technology advances rapidly, infusing itself throughout society and leading to widespread conflict and displacement as machines rapidly replace humans in a sweeping revolution. The reality is that today’s AI revolution is happening far more slowly and relatively silently, with algorithms often displacing traditional human tasks in less visible tasks behind the scenes, while the pace of this revolution is far slower than the public hype around AI might suggest.
The Hollywood version of the AI revolution typically revolves around a singular breakthrough in AI technology that leads to exponential growth in machine intelligence, displacing humans and upending the societal structure until, in the blink of an eye, algorithms are in charge.
Today’s reality is far more mundane. Lacking the “singularity” breakthrough that would free machines from their human creators, today’s relatively primitive correlative algorithms must be purpose-built for each task, regularly refreshed as their models stagnate and carefully wrapped in protective layers to safeguard surrounding systems from their brittleness.
The end result is that far from general purpose learning systems that can be dropped in to replace any human, deep learning algorithms have become what amount to bespoke suits, hand crafted from a common cloth, but tailored for each individual application and requiring regular adjustments as the underlying inputs change over time.
This isn’t to say the AI revolution has stalled, but rather that its roll-out is far from the explosive public spectacle that defined the rapid rise of the Web in its early days.
Perhaps the biggest difference is that while the Web was a consumer-facing technology, creating and deploying even relatively simplistic deep learning solutions today requires a level of technical expertise not widely available among a typical company’s programmer ranks. Those capable of wielding state-of-the-art systems or even advancing the field itself are an even greater rarity.
This has dramatically slowed the introduction of deep learning systems within the commercial space, since companies must prioritize how to apply their small number of specialists, much as data scientists were once rare assets in the corporate world.
The bespoke nature of these tools means each deployment requires a development cycle all to itself, much as the rollout of desktop computers within the enterprise once required a similarly slow and methodological introduction. Whereas today a data scientist can whip up a quick map or network visualization in a few tens of minutes, building, tuning, testing and deploying a robust deep learning solution represents a considerable undertaking.
Solutions like transfer learning and automatic model construction can help automate many of these pieces, but still require considerable application-specific investments in data annotation and curation.
The brittleness of today’s systems means companies must also devote considerable resources towards understanding the situations under which they may fail and constructing the necessary cushioning to minimize the impact of such failures on the applications themselves. This can take the form of hand-coded rulesets for the most mission-critical decisions or combining deep learning and classical models, with special handling of cases in which the two diverge beyond a certain threshold.
Despite these limitations, deep learning is finding no shortage of applications in the enterprise, automating many tasks that had historically been strongly resistant to codification due to their noisy data, complex patterns or multimedia source data.
Yet these applications are typically located outside of the limelight. In contrast to the splashy research demonstrations playing video games or teaching robots how to learn to walk, production deployments today tend to be far more mundane and located in less visible places, from image filtering to chat bots to routing systems. Each deployment displaces human workers that once filled those jobs or reduces the need to hire new workers, but its introduction is typically little publicized and little noticed outside those it immediately effects.
Interestingly, while companies tend to proudly tout their overall corporate adoption of deep learning, outside of a small realm of applications like voice recognition and digital assistants, users are rarely made aware that they are interacting with a deep learning algorithm. Their only hint of an automated provenance may be the fact that the “person” on the other end seems to be remarkably adept at some tasks but comically incapable of others or that a backend process that formerly took days now finishes within seconds.
In the end, we speak of an “AI revolution” upending society overnight. Yet if we look a bit closer it seems this “revolution” is a far slower, steadier and largely silent “evolution.”