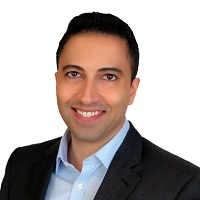
In this special guest feature, Dr. Eli David, PhD, Co-founder of DeepCube, highlights the 3 questions driving the future of AI. Dr. David is a leading expert in the field of computational intelligence, specializing in deep learning and evolutionary computation. DeepCube, the first software-based inference accelerator, and Deep Instinct, the first company to apply deep learning to cybersecurity and was selected by the World Economic Forum as a Technology Pioneer. Dr. David has published over fifty papers in leading artificial intelligence journals and conferences, mostly focusing on applications of deep learning and genetic algorithms in various real-world domains. For the past fifteen years, he has been teaching courses on deep learning and evolutionary computation, in addition to supervising the research of graduate students in these fields. He has also served in numerous capacities successfully designing, implementing, and leading deep learning based projects in real-world environments.
AI adoption has become a key topic of focus as organizations look to use the technology to drive efficiencies and realize ROI. With that, AI is finding its way into more industries and a growing number of companies already experience the benefits. However, despite periods of significant advancement over the last decade, many challenges remain, hindering widespread adoption and implementation.
From challenges in computational requirements, to high costs, and the technical limitations of bringing deep learning models to the edge, AI still has significant progress to be made in order to realize real-world deployments at scale. In looking to address these challenges, three overarching questions will serve as a bellwether for the future of AI.
<!–
<!–
–>
How do we bring AI applications to the real-world?
Deep learning, the key driver of most AI advancement the last several years, draws from how the human brain operates to process significant amounts of data for use in decision-making.
Deep learning has produced incredible lab results; however, these models are incredibly large and require considerable processing power, which has confined them to labs and the cloud. In turn, this limits the potential real-world use cases and stymies widespread adoption.
While cloud deployments present a viable solution for some use-cases like smart home devices, it brings issues of latency, connectivity, privacy, and high costs. If deep learning is deployed in the cloud, the edge device must have constant internet connectivity and be dependent on the speed in which data can be processed and transferred to and from the cloud. In many cases, this makes it a non-starter.
To overcome the deployment problem, edge deployment is necessary. But, how do we bring deep learning to the edge? We must shrink the models to a compatible level, bringing us to the second question.
How can we shrink the computational requirements and size of deep learning models?
Realizing edge deployments begins with reimagining the model training process, drawing inspiration from early stage human brain development.
In early childhood, we have the greatest number of synapses – connections through which neurons communicate – that we will have in our lifetime. Up until our late teenage years, our brain is constantly removing redundant connections and becoming sparser, with connections themselves learning rapidly, and the entire structure of our brain continuously modifying.
During the deep learning training process, we can attempt to mimic this and sparsify the model to find a balance between the size of the model and its accuracy. By undergoing pruning in the training stage, when the model is most receptive to restructuring, results can be drastically improved and accuracy maintained.
The resulting model can be lightweight with significant speed improvement and memory reduction, allowing for an efficient deployment on intelligent edge devices and enabling real-time, autonomous decision making.
What will the future of AI deployments look like?
Progress is being made to overcome these obstacles, but what is the end game? With two possible deployment strategies of deep learning technology – in the cloud and at the edge – which deployment method should be adopted?
Cloud deployments allow AI to benefit from the power of high-performance computing systems, but bring about privacy concerns and limitations due to latency, bandwidth, and connectivity.
AI at the edge alleviates some of the privacy and bandwidth concerns as well as latency constraints. Moreover, it provides significant improvements to speed, power, and memory consumption, which can cut costs and limit the environmental impact. However, it sacrifices computational power and the ability to sync data across devices.
The benefits of one cannot be fully replaced by the other; and therefore, the most impactful, real-world AI deployments will be those that take a hybrid approach: in the cloud and at the edge.
A hybrid approach would allow models to be retrained with cross-device data for continuous improvement in the cloud while maintaining the speed, efficiency, and security of edge deployment.
Workflows can be developed to maximize efficiency and scalability; specifically, by identifying use cases in which decisions must be made at the edge, in real-time, complemented by scenarios where processing can take place in the cloud for long-term analysis and improvement.
Take autonomous vehicles as an example. If a car cannot act until data has been sent to the cloud and processed, then it will not be able to react and make decisions quickly enough to ensure safety. However, without the associated cloud deployment, insights cannot be combined with data gathered from other models for algorithm improvement.
Realizing AI’s future
While the technology to bring deep learning models to the edge is in place, we are leaps away from bringing it out of the lab and onto real-world deployments at scale. However, the rapid rate at which deep learning is being studied and adapted may culminate into the emergence of real-world deployment. The exponential advancement of AI research will eventually allow businesses to effectively deploy the technology and take deep learning models out of the lab. For any business looking to expand offerings and capabilities or streamline efficiencies with deep learning these three questions will set the tone for 2021 and beyond.
Sign up for the free insideBIGDATA newsletter.
Join us on Twitter: @InsideBigData1 – https://twitter.com/InsideBigData1
You must be logged in to post a comment.