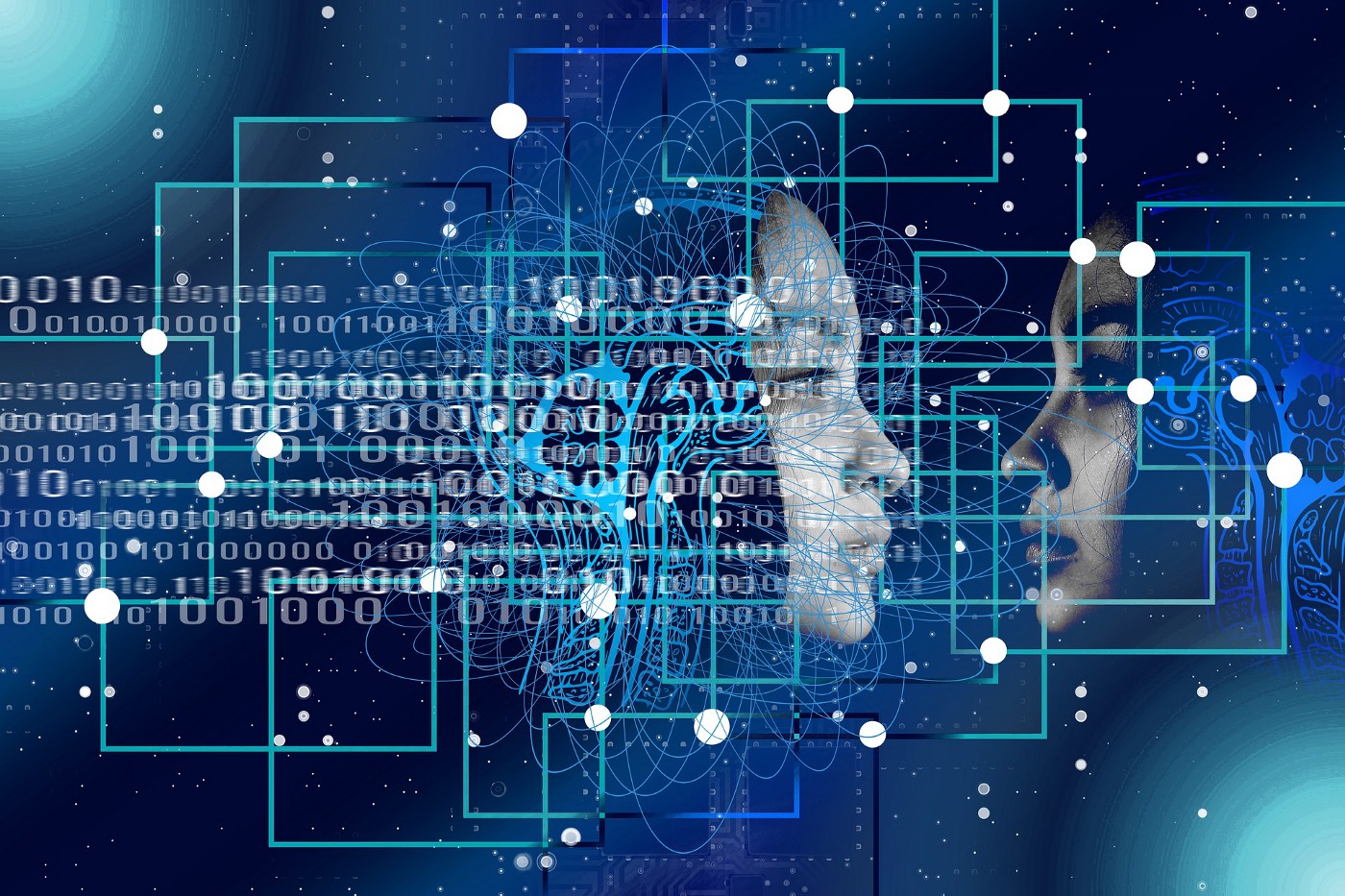
Is model operationalization really necessary? Every single day, companies spend millions of dollars on data scientists, software engineers and AI applications. Most of these companies have no strategy for making the most of their investment. They don’t know how to leverage their technology investment for maximum impact on revenue or profits.
Artificial Intelligence is a hotter topic today than ever. From self-driving cars to personal assistants, AI is slowly making its way into our daily lives. Artificial Intelligence (AI) is an area of computer science that studies the possibility of thinking computers and machines.
What’s more, it’s no longer a theory but a reality in many ways. There are already many applications in place that have been developed with the help of AI, including business applications.
The past decade has seen an explosion of applications for artificial intelligence, machine learning, and deep learning. This has led to advances in a wide range of application domains, including document classification and processing, natural language understanding, and bioinformatics. A growing number of highly effective methods for speech processing and image classification are being applied successfully to robotics and computer vision. We are witnessing exponential growth in the use of deep learning in web search algorithms, translators, speech recognition, image and photo classifiers. Furthermore, artificial intelligence has allowed us to automate repetitive tasks with little or no human intervention.
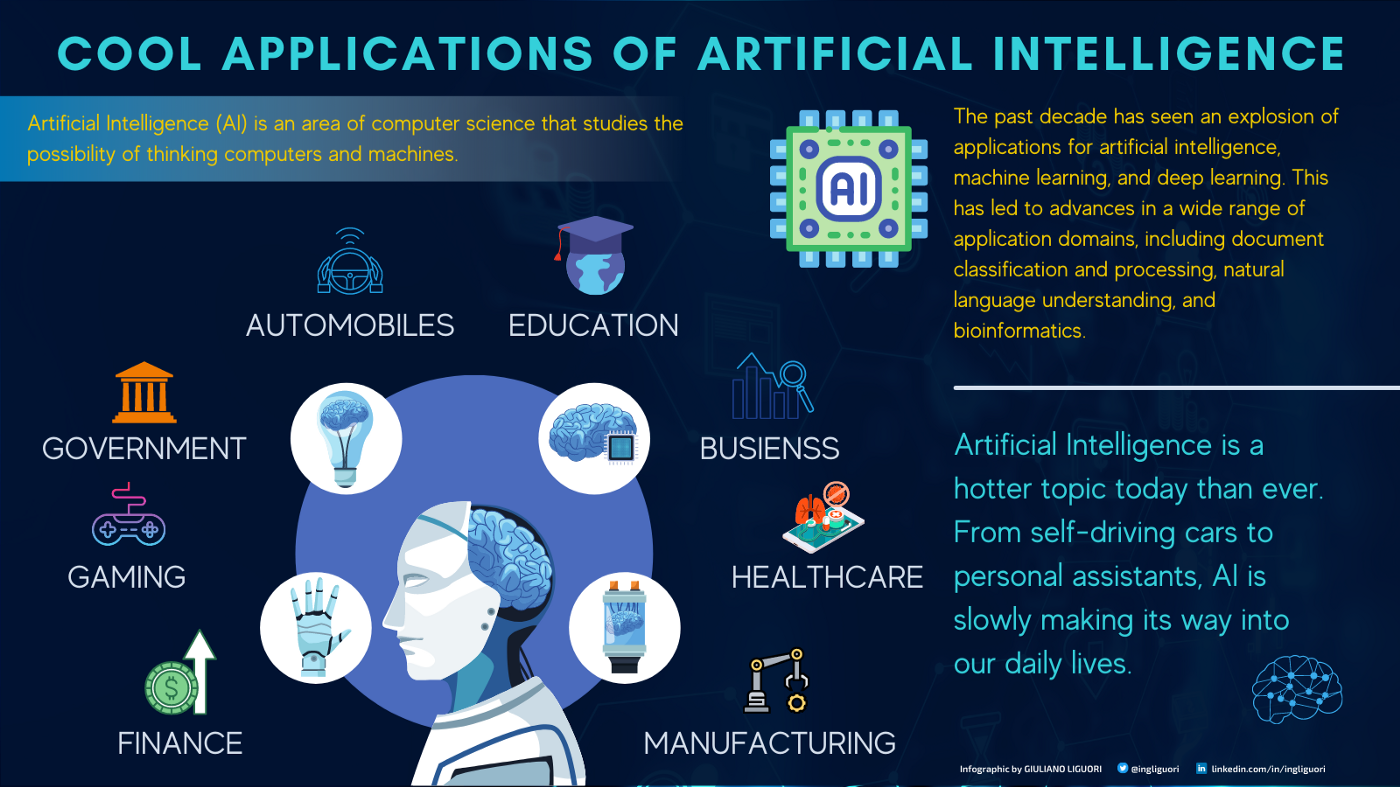
There has been a lot of talks lately about the promise of value linked to the potential of artificial intelligence. However, the return on investment in AI is proving difficult for many organizations, and worse, they see themselves falling behind others who have overcome the challenge. Often, the discussion revolved around technologies that were fundamental and enabling, but not enough to generate business results. For most organizations, interest in AI has initially been focused on the delivery of analytical and machine learning models as artifacts in this first phase of its exploitation. On the other side, the aspect of their integration with IT and business delivery processes has been overlooked, that is, the aspect of their “operationalization”.
The latter aspect is fundamental to reduce the “time-to-value”, considering that it takes months to integrate an AI model within a business workflow and to achieve the first benefits.
There are many reasons for this, but a key one is that AI requires different skills than traditional software development. Most companies have data scientists who can create models and algorithms that power their artificial intelligence systems, but few have technical staff who can create and maintain the software infrastructure that enables these models to run and large-scale algorithms. At a high level, the software infrastructure required for AI may seem similar to that required for traditional software development. But at a lower level, it requires different skills and thinking. The people best suited to build this infrastructure are those with experience in both technology, process management and a strategic vision of business and innovation.
The reasons behind the complexity are different. The lack of security and privacy, inadequate volume and quality of data, accessibility challenges, limited understanding of the use cases and related benefits, insufficient internal skills, to name just a few. The real risk of such a situation is to create skepticism on the part of important stakeholders about the benefits and transformative impact of AI on their organization. The enthusiasm for AI may fade and AI will be perceived as just another technology, rather than something that can fundamentally change the way organizations operate.
What can we do to handle this complexity? Data is of course the common element underlying all of the interdependencies. Although it is necessary to work on technologies, it is also necessary to consider the aspect of work practices and operating models. All that is necessary in order to integrate data and AI in an industrialized way into operations and business processes.
ModelOps (AI Model Operationalization) is undoubtedly at the heart of any AI strategy. ModelOps is a holistic approach to making predictive analytics and machine learning workflows operational. Teams benefit from putting these workflows into action, making the most of them while minimizing wasted time on repetitive tasks, allowing them to focus on what really matters. Gartner defines ModelOps, which are practices whose goal is to automate a common set of operations that arise in data science projects, such as model training pipelines, version control, data management, experiment monitoring, testing, and distribution. It aims to operationalize all predictive analytics, machine learning, and artificial intelligence models. It helps in building Automated Test Procedures that detect coding errors along the project delivery pipeline.
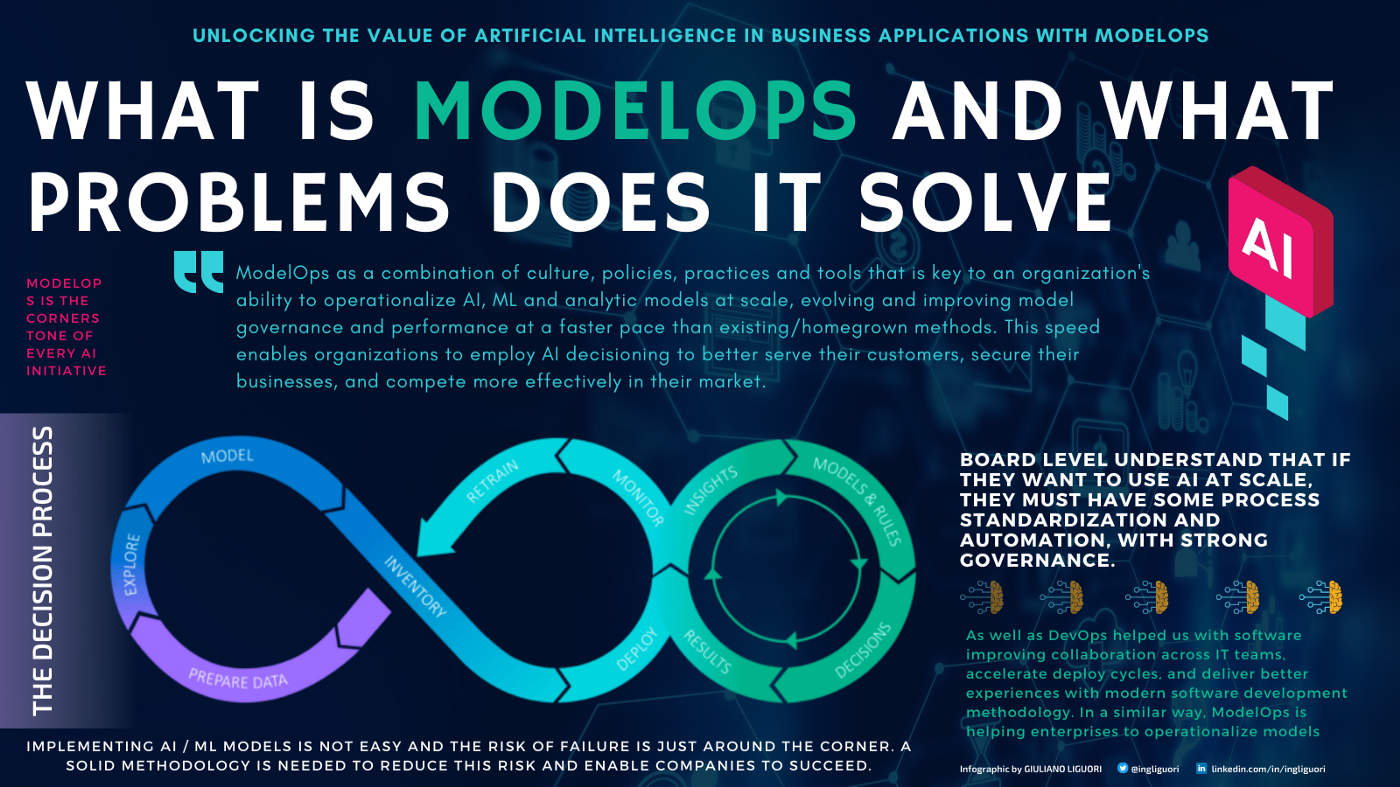
Organizations that are making huge investments to develop AI models can do a lot to improve their efficiency. They can optimize their processes, streamline their activities, and increase their margins by shifting from traditional manual practices to industry-best model operations. A ModelOps platform like ModelOp Center automates all aspects of model operations, no matter the type of model, how it is developed, or where it is run. Automation has a number of benefits. It improves decision-making to increase lead times and maximize production rates and capacity. It helps businesses plan better and cut costs by minimizing personnel costs. It helps capture data that otherwise would have been lost with manual methods.
ModelOp Center is an innovative solution that automates all aspects of model operations, regardless of the type of model, how developed, or where the model is run. Using ModelOp Center’s platform, organizations can easily and effectively automate and manage model operational processes. In addition to delivering significant operational efficiency improvements for their internal teams, ModelOp Center’s clients also benefit from reduced costs and improved control over model operations.
Customers can choose to install ModelOp Center in their environment, either on-premises or in their private cloud whether AWS, Azure, or Google Cloud Platform (GCP).
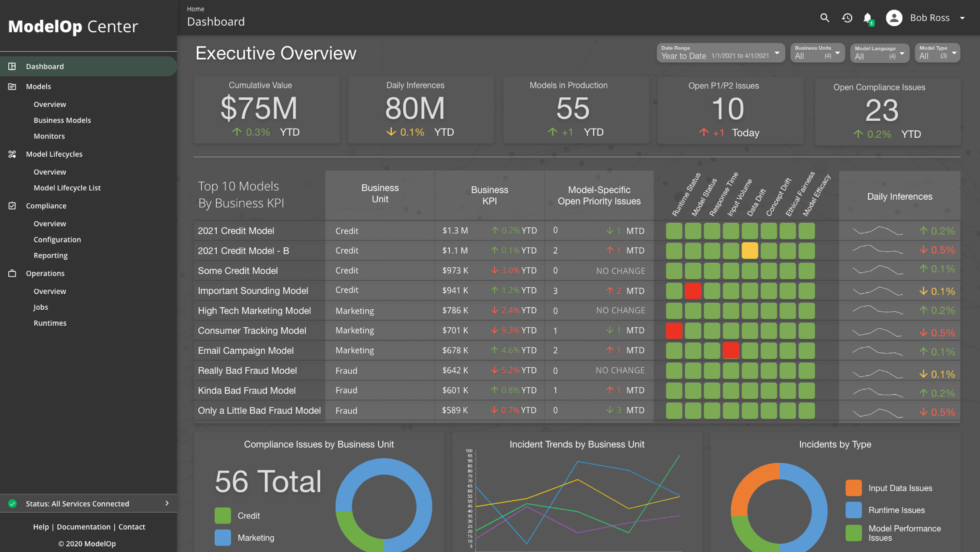
The ModelOps for Dummies is a good book to read.
Taking the stance that no one reading this book is a dummy, not before, nor after. This book is for Board and C-levels Executives that want to survive and thrive in the coming model-driven. This book is not for data scientists it is aimed at leaders in charge of innovation management, that are responsible for transforming their organization into a model-driven enterprise. They don’t create the AI models; they just use them in business to do. They are the people who have the responsibility to provide value to their organizations by proving the actual ROI from any AI project. This book is intended for those who want to protect their business from model risk and compliance issues.
There is a lot of reading about Python, C ++, and how to train AI/ML models. But everyone seems to be discovering the real issue in AI: how businesses can put these things into operation and scale. This is the real reason why I recommend you read this book because it focuses on the topic of business and risk management.
In my opinion, ModelOps for Dummies is a must-read for leaders that aim to:
- measuring their return on investment from AI;
- capturing a 360 view of their models across the entire enterprise;
- managing model risk and compliance issues.
This book is unlike any other book on AI. It’s written by a business executive for business executives and easily navigates through what is typically a very technical topic and is not intended for the data scientist.

You can get your free copy here https://bit.ly/MdlOpsB
- ModelOps is a capability that lies at the center of any organizations’ enterprise AI strategy. It is a technology that can be used to converge and centralize various AI artifacts, platforms, and solutions while ensuring scalability and governance.
- By integrating business processes into ModelOp Center, not only do we solve deployment, monitoring, and governance of models, but we also create a bridge between Operations, IT, and Data Science.
- Whether you’re a seasoned veteran in AI or ML, or just beginning to explore the possibilities, the book ModelOps for Dummies will teach you how to leverage ModelOps and make your organization more competitive.
Also read Including ModelOps in your AI strategy