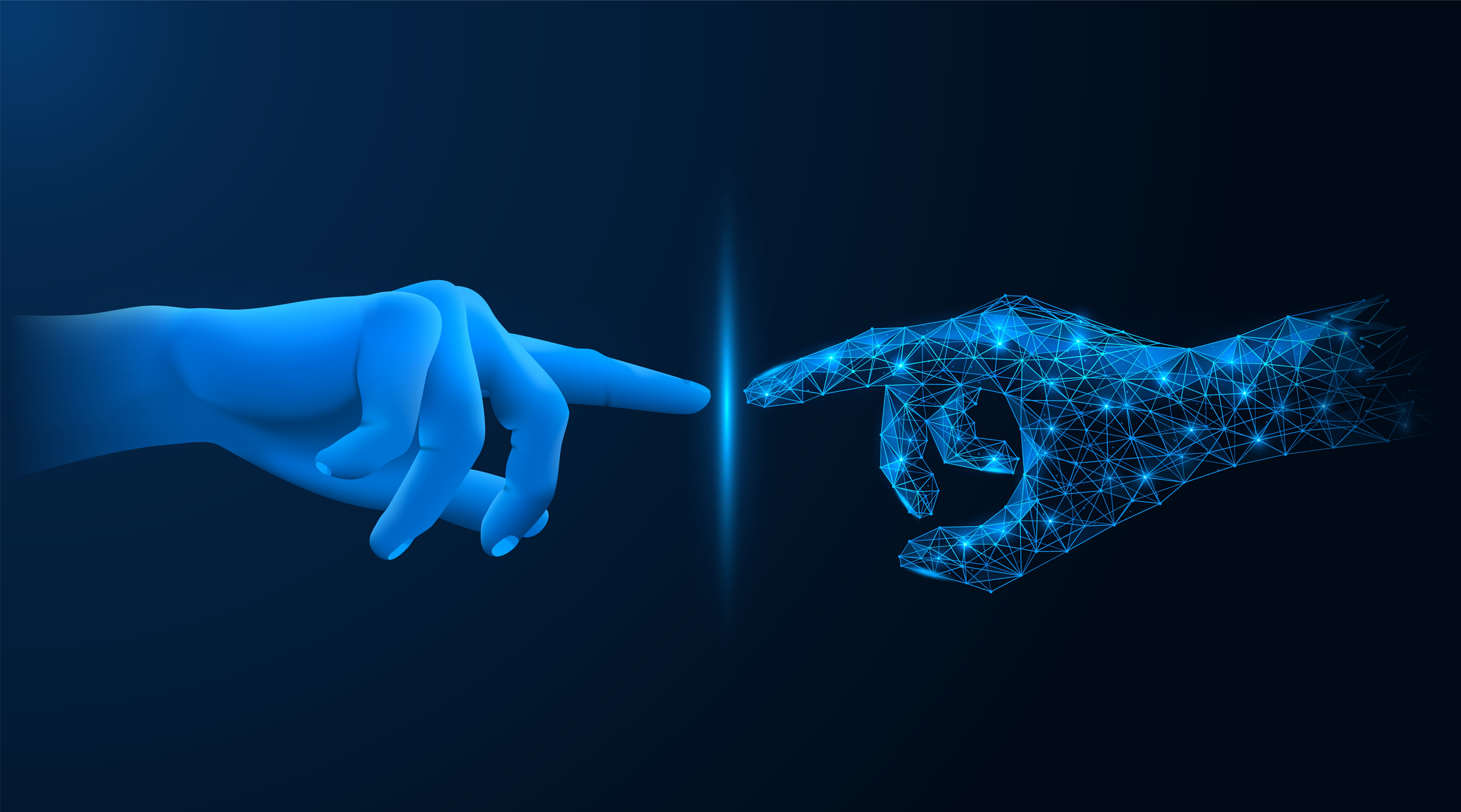
istock
As someone who is passionate about the transformative power of technology, it is fascinating to see intelligent computing – in all its various guises – bridge the schism between fantasy and reality. Organisations the world over are in the process of establishing where and how these advancements can add value and edge them closer to their goals. The excitement is palpable.
However, it is important that this excitement does not blind us to the dangers, propelling us ahead without having taken the right preparatory steps or without understanding the challenges that will be encountered along the way.
Preparing for an artificial intelligence (AI)-fueled future, one where we can enjoy the clear benefits the technology brings while also the mitigating risks, requires more than one article. This first article emphasizes data as the ‘foundation-stone’ of AI-based initiatives.
Establishing a Data Foundation
The shift away from ‘Software 1.0’ where applications have been based on hard-coded rules has begun and the ‘Software 2.0’ era is upon us. Software development, once solely the domain of human programmers, is now increasingly the by-product of data being carefully selected, ingested, and analysed by machine learning (ML) systems in a recurrent cycle. In this new era the role of humans in the development process also changes as they morph from being software programmers to becoming ‘data producers’ and ‘data curators’ – tasked with ensuring the quality of the input.
This would be straightforward task were it not for the fact that, during the digital-era, there has been an explosion of data – collected and stored everywhere – much of it poorly governed, ill-understood, and irrelevant. Data lakes have been amassed during a time when organisations have been pre-occupied with ‘infrastructure-first transformation’ initiatives. And, while it may be useful to digitize business processes, unburden yourself from siloed multi-generational IT, and drive cloud-first mandates, it will only get you so far on the transformation continuum.
Data Centricity
Forward-thinking transformation leaders have realised that more focus needs to be placed on ‘data-centric value creation’ and have made this the pre-eminent organising principle in their organisations. “Data-first,” as a basis for technology and other critical investment decisions, can:
- Spur new operating models that help them differentiate and grow
- Create ‘hyper-personalised’ digital moments and experiences that drive loyalty
- Improve foresight and expand predictive capabilities
These leaders are doing so not just to help them fully embrace the digital ‘now,’ but to prepare for and capitalise on the AI-fuelled digital ‘next.’
Exposing the Blindspot
There is little doubt that the next wave of technology, driven by greater automation and computational intelligence, will rely on data more than any preceding era. To take full advantage of these advancements data must be:
- Well understood and well organised
- Continually analysed for relevance and cleansed
- Sensibly located where it can add most value and be accessed in a frictionless, cost-effective way
- Carefully selected to drive the optimal business outcomes
- Tightly governed and regulated such that it is compliant and ethically sound
To overlook or downplay the importance of any of these considerations is to potentially build your AI future on pillars of sand.
There is evidence to suggest that there is a blind spot when it comes to data in the AI context. Many organisations focus too heavily on fine tuning their computational models in their pursuit of ‘quick-wins.’ However, contrary to popular belief, AI success is not about tweaking and recalibrating models, it’s about tweaking data, continually.
Once built, the computational models should remain relatively static. Most industry experts believe it is data availability, quality, and understanding that are the biggest determinants of success in AI. Without them an organisations’ AI exploits carry significant risk, particularly due to the triple-threats of data bias, mis-labelling, and poor selection.
Despite soundings on this from leading thinkers such as Andrew Ng, the AI community remains largely oblivious to the important data management capabilities, practices, and – importantly – the tools that ensure the success of AI development and deployment.
Addressing the Challenge
Data-centric AI is evolving, and should include relevant data management disciplines, techniques, and skills, such as data quality, data integration, and data governance, which are foundational capabilities for scaling AI. Further, data management activities don’t end once the AI model has been developed. To support this, and to allow for malleability in the ways that data is managed, HPE has launched a new initiative called Dataspaces, a powerful cloud-agnostic digital services platform aimed at putting more control into the hands of data producers and curators as they build intelligent systems.
Addressing, head on, the data gravity and compliance considerations that exist for critical datasets, Dataspaces gives data producers and consumers frictionless access to the data they need, when they need it, supporting better integration, discovery, and access, enhanced collaboration, and improved governance to boot.
This means that organisations can finally leverage an ecosystem of AI-centric data management tools that combine both traditional and new capabilities to prepare the enterprise for success in the era of decision intelligence. A great example of this is Novartis.
Recommendations for Data and AI Leaders
In summary, in order to ensure that AI programs are a success from the outset, organisations should take the following data-related steps:
- Formalise both ‘data-centric AI’ and ‘AI-centric data’ as part of data management strategy with metadata and data fabric as key foundational components.
- Set policy guardrails that include mandatory minimums about ‘data fitness’ for AI, to protect against bias, mislabelling, or irrelevance.
- Define the appropriate formats, tools, and metrics for AI-centric data as early as possible, preventing the need to reconcile multiple data approaches as AI scales.
- Seek diversity of data, algorithms, and people within the AI supply chain to ensure value is realised and ethical approaches are taken.
- Establish roles and responsibilities to manage data in support of AI, leveraging AI engineering and data management expertise (internal and external) and approaches to support ongoing deployment and production uses of AI.
The next article will focus on how to increase the transparency and ‘explainability’ of AI systems in order to effectively remove bias within the data or the computational models – reducing the inherent risk in the process.
To learn more, visit HPE.
____________________________________
About Andrew P. Ayres MBA
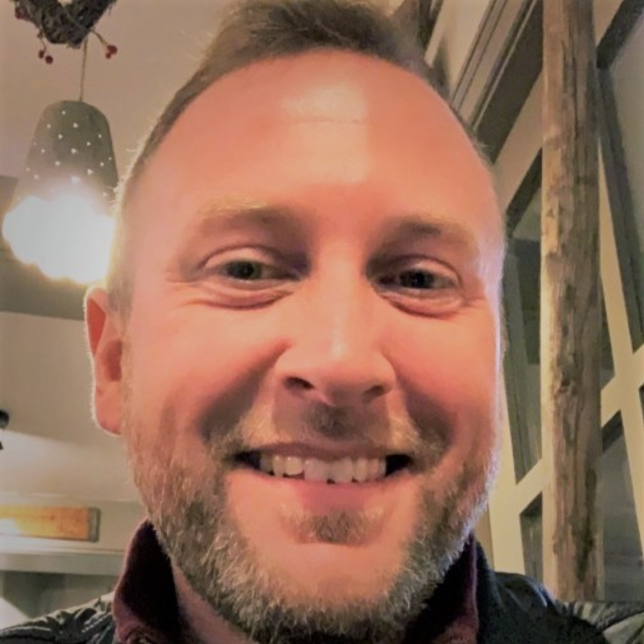
Following a successful career with Gartner and Micro Focus – Andrew is now a Senior Specialist within HPE’s Enterprise Services practice in the UK – focusing on the Financial Services & Insurance industry. As a subject-matter expert in digital transformation, data-centric modernisation, cloud computing and artificial intelligence – Andrew helps bring together the best of HPE’s capabilities to ensure clients are future-fit and ready to meet the ever-changing needs of their customers.
Andrew holds an MBA from Manchester Business School and is currently a PhD Researcher at Manchester Metropolitan University and his thesis centres on how Banks can govern against the risks posed by Artificial Intelligence in the context of their High Frequency Trading operations.
He is based in Manchester, UK
You must be logged in to post a comment.