One issue in robotics is enabling the robot to detect danger. That’s harder than it might seem; it involves evaluating uncertainties. In their open access paper, a Harvey Mudd College research group describes the situation in dramatic terms by asking readers to picture a dilemma:
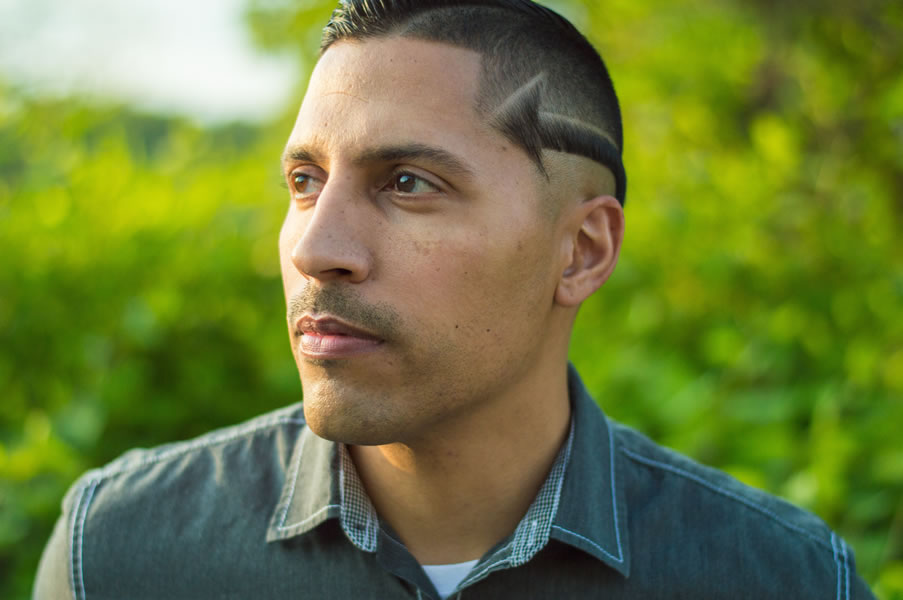
Imagine a wealthy individual has announced they have hidden a large sum of money in an abandoned mine. You feel particularly adventurous and visit the mine in search of treasure. Approaching one of the mine’s many entrances, your excitement plummets as you notice the hazardous conditions. The precarious wooden floor planks separating you from a 50-foot drop are worn and rotted. Trails of crumbling rock intermittently fall from the roof and walls, indicating a potential cave-in at any time. You slowly realize this may not be accidental; perhaps the owner of the mine intended to make the situation hopelessly dangerous. As you survey the space with increasing skepticism, you notice some strange beam-and-rope structures attached to a few of the platforms—their trap-like appearance sets off additional red flags. Weighing your safety against the possibility of reward, you decide the perilous quest is not worth the risk.
C. Hom et al., “The Gopher’s Gambit: Survival Advantages of Artifact-Based Intention Perception.” 13th International Conference on Agents and Artificial Intelligence (ICAART 2021), Online, Feb 4–6, 2021.
The problem with going ahead may seem “obvious” to humans. But conveying that “red flag” sense to a robot is another matter. The robot has no independent life experience to rely on.
Can we build in an awareness of potential danger? The Harvey Mudd team, led by assistant professor George Montañez, decided to use digital gophers instead of humans (gophers are simpler) to test the possibilities for programming robots to detect the intentions behind circumstances (as in “it looks like a trap…”):
The computer simulation created virtual gophers and “tested whether intention could be perceived through artifacts, namely, structures in an environment that could potentially be traps,” Montanez added. Further, the study looked at whether the virtual gopher’s “knowledge of whether the structures were intended or accidental could improve survival rates for artificial agents (in our case, artificial gophers).”
Marjorie Hecht, “Harvey Mudd’s virtual gopher study examines how detection of deliberate traps improves survival” at Current Science Daily (November 15, 2021) The paper is open access.
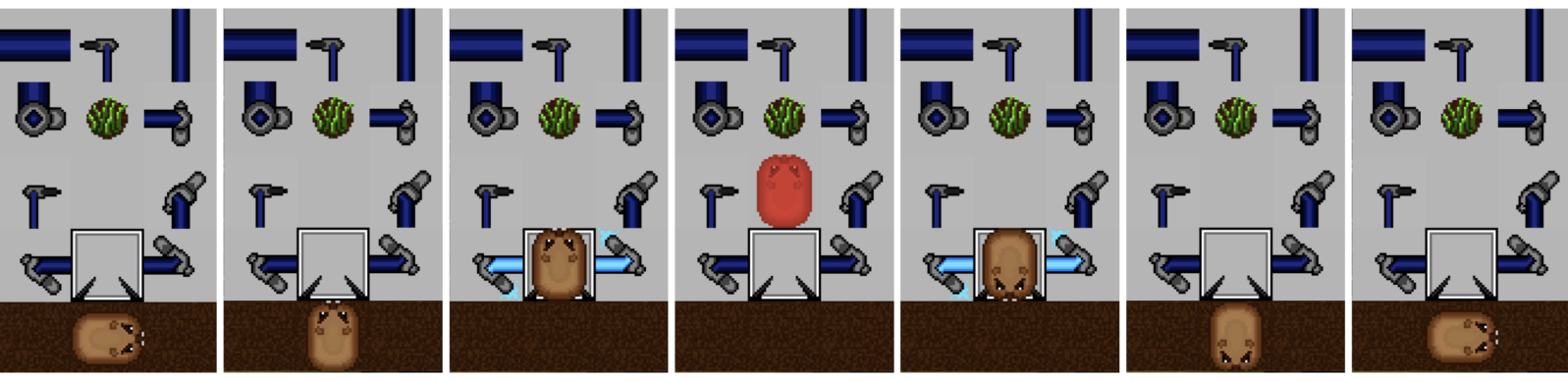
It’s not a straightforward decision: The way the program works is that if the “gopher” avoids too many possible food sources. it will starve but if it lunges into too many structures in pursuit of food, it will get trapped.
And the result of coding gophers to have — or not have — intention perception?
The researchers found that “those gophers who were able to accurately determine whether the structures were designed with the intent to harm allowed the intention-perception gophers to avoid many lethal traps and, therefore, live longer.
Marjorie Hecht, “Harvey Mudd’s virtual gopher study examines how detection of deliberate traps improves survival” at Current Science Daily (November 15, 2021) The paper is open access.
The advantages were more significant than expected. From the paper: “We find that gophers possessing the ability to perceive intention have significantly better survival outcomes than those without intention perception in most of the cases evaluated.”
Intention perception, if it can be generalized, might prevent complex robotic devices from being taken over or destroyed by comparatively simple hacks.
The team plans two more papers, focusing on the advantages of direct vs. indirect intention detection.
Natural gopher:
You must be logged in to post a comment.